People
John Sous
Assistant Professor of Applied Physics; Member of the Energy Sciences Institute
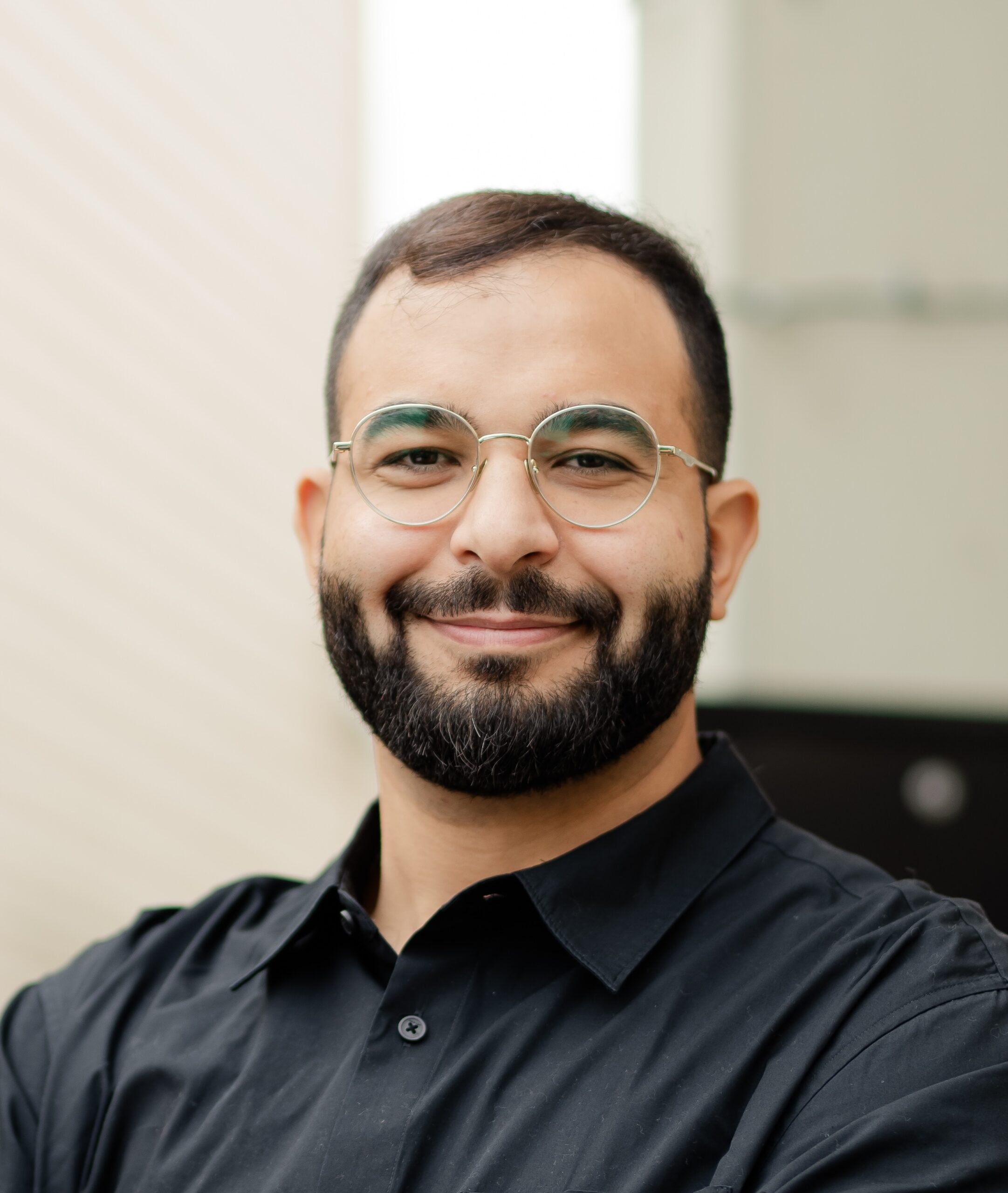
Professor Sous’s research focuses on the study of statistical systems with large number of degrees of freedom in which correlations act to stabilize novel behavior. Examples include quantum materials, nonlinear dynamical systems and models of neural learning. Professor Sous obtained his Ph.D. in Physics from the University of British Columbia. After that he spent three years at Columbia University and a year at Stanford University as a postdoc. He joined Yale in 2024 after spending a year on the faculty at UCSD.
What do you do with Data Science?
I am a scientist with an interdisciplinary background who made significant scientific contributions to the physics of quantum matter. Some of my works advanced and employed state-of-the-art computational and data-driven approaches such as tensor networks (also known as tensor trains) and Monte Carlo. I truly believe we are at a pivotal moment in history where current discoveries in AI are occurring at a rate that supersedes our ability to manage and control. This is why I believe it is important that researchers attack the problem of interpretability of AI from different angles. Given my background as a physicist who likes to understand “why things behave in the way they do”, my work takes a physics (mechanistic) perspective on AI models. This is commonly known as mechanistic interpretability, which, in a nutshell, can be viewed as the task of identifying (not necessarily analytically) simple models which demonstrate the mechanism behind a functionality demonstrated in deeper models. This research has the potential to lead to AI alignment and safety as well as advanced capabilities of practical usefulness, such as AI models for reasoning and for science. Examples of topics I am currently interested in include understanding composition in transformers in the context of learned mathematical operations, score-matching energy-based models, amongst others.